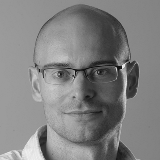
Pieter Abbeel
La nomination
Spécialiste-conseil
Apprentissage automatique, apprentissage biologique
Connect
À Propos
Pieter Abbeel travaille dans le domaine de l’apprentissage automatique et de la robotique.
Plus particulièrement, il se penche sur l’apprentissage par la pratique (faire en sorte que les robots apprennent des gens) et l’apprentissage par renforcement (comment faire pour que les robots apprennent à tâtonnement), et souhaite accélérer l’acquisition de connaissances par l’entremise de l’apprendre-à-apprendre. Les robots d’Abbeel ont appris à réaliser des voltiges aériennes complexes d’hélicoptère, à faire des nœuds et à organiser la lessive. Son groupe de recherche a créé l’apprentissage profond par renforcement pour la robotique, y compris l’acquisition de compétences visuomotrices et le déplacement simulé.
Prix
- Prix du meilleur article, lauréat ou finaliste (NIPS, CIFAR (quatre fois), ICML)
- Presidential Early Career Award for Scientists and Engineers (PECASE)
- Prix IEEE Robotics and Automation Society pour chercheur en début de carrière
- Prix du programme des jeunes chercheurs: ONR, AFOSR, Darpa, NSF
- Prix Dick Volz pour la meilleure thèse de doctorat en robotique et en automatisation aux États-Unis
Publications Pertinentes
- Duan, Y. et coll. « One-shot Imitation Learning. » Article présenté à la conférence Neural Information Processing Systems (NIPS), 2017.
- Tobin, J. et coll. « Domain Randomization for Transferring Deep Neural Networks from Simulation to the Real World. » Dans Proceedings of the 30th IEEE/RSJ International Conference on Intelligent Robots and Systems (IROS), Vancouver, octobre 2017.
- Finn, C., P. Abbeel et S. Levine. « Model-Agnostic Meta-Learning for Fast Adaptation of Deep Networks. » Dans Proceedings of the International Conference on Machine Learning (ICML), Sydney, août 2017.
- Chen, X. et coll. « InfoGAN: Interpretable Representation Learning by Information Maximizing Generative Adversarial Nets. » Article présenté à la conférence Neural Information Processing Systems (NIPS), 2016.
- Schulman, J. et coll. « Trust Region Policy Optimization. » Dans Proceedings of the 32nd International Conference on Machine Learning (ICML), 2015.