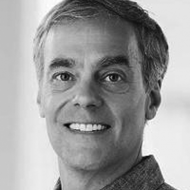
Richard Zemel
Appointment
Associate Fellow
Canada CIFAR AI Chair
Learning in Machines & Brains
Pan-Canadian AI Strategy
About
Appointed Canada CIFAR AI Chair – 2019
Richard Zemel is a Canada CIFAR AI Chair at the Vector Institute, a CIFAR associate fellow of the Learning in Machines & Brains program, a professor in the department of computer science at the University of Toronto, and a research scientist at Google Brain. He is a research director at the Vector Institute, the Google/NSERC industrial research chair in machine learning, and the chief scientist for machine learning at the Creative Destruction Lab at the Rotman School of Business. Zemel is also the co-founder of SmartFinance, a financial technology start-up specializing in data enrichment and natural language processing.
Zemel’s research contributions include foundational work on systems that learn useful representations of data without any supervision; methods for learning to rank and recommend items; and machine learning systems for automatic captioning and answering questions about images.
Awards
- Industrial Research Chair in Machine Learning, NSERC, 2018
- Pioneers of AI, NVIDIA, 2016
- Discovery Accelerator Award, NSERC, 2009, 2014
- Dean's Excellence Award, University of Toronto, 2005-2008,2011, 2013, 2014
Relevant Publications
Klys, J., Snell, J., & Zemel, R. (2018). Learning latent subspaces in variational autoencoders. In Advances in Neural Information Processing Systems (pp. 6444-6454).
Madras, D., Creager, E., Pitassi, T., & Zemel, R. (2018). Learning adversarially fair and transferable representations. arXiv preprint arXiv:1802.06309.
Snell, J., Swersky, K., & Zemel, R. (2017). Prototypical networks for few-shot learning. In Advances in neural information processing systems (pp. 4077-4087).
Li, Y., Tarlow, D., Brockschmidt, M., & Zemel, R. (2015). Gated graph sequence neural networks. arXiv preprint arXiv:1511.05493.
Zemel, R., Wu, Y., Swersky, K., Pitassi, T., & Dwork, C. (2013, February). Learning fair representations. In International Conference on Machine Learning (pp. 325-333).
Support Us
CIFAR is a registered charitable organization supported by the governments of Canada and Quebec, as well as foundations, individuals, corporations and Canadian and international partner organizations.