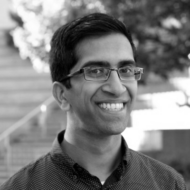
Gautam Kamath
About
Appointed Canada CIFAR AI Chair – 2023
Gautam Kamath’s research focuses on considerations related to trustworthy machine learning, particularly concerns of privacy and robustness. For example, how can we ensure that a machine learning model doesn’t leak sensitive information about its training data? Can we guarantee that a machine learning model is protected against interference by malicious actors? He tries to understand the impact of these constraints at a fundamental theoretical level, as well as develop practical methods for training models which are privacy-preserving, robust, or more generally, suitable for use in the wild.
Awards
- Waterloo Faculty of Mathematics Golden Jubilee Research Excellence Award, 2023
- CCS Best Reviewer, ACM SIGSAC (2021)
- Discovery Accelerator Supplement, NSERC (2020)
- Akamai Presidential Fellow, MIT (2012)
- Computer Science Prize for Academic Excellence, Cornell University (2012)
- STOC Best Student Presentation, ACM SIGACT (2012)
Relevant Publications
- Hopkins, S. B., Kamath, G., Majid, M., & Naryanan, S. (2023). Robustness Implies Privacy in Statistical Estimation. Proceedings of the 55th Annual ACM Symposium on the Theory of Computing, STOC ’23.
- Yu, D., Naik, S., Backurs, A., Gopi, S., Inan, H. A., Kamath, G., Kulkarni, J., Lee, Y. T., Manoel, A., Wutschitz, L., Yekhanin, S., & Zhang, H. (2022). Differentially private fine-tuning of language models. Proceedings of the 10th International Conference on Learning Representations, ICLR ’22.
- Canonne, C. L., Kamath, G., & Steinke, T. (2022). The discrete Gaussian for differential privacy. Journal of Privacy and Confidentiality, 12(1).
- Sekhari, A., Acharya, J., Kamath, G., Suresh, & A. T., (2021). Remember What You Want to Forget: Algorithms for Machine Unlearning. Advances in Neural Information Processing Systems 34, NeurIPS ‘21.
- Diakonikolas, I., Kamath, G., Kane, D. M, Li, J., Moitra, A., & Stewart, A. (2019). Robust estimators in high-dimensions without the computational intractability. SIAM Journal on Computing, 48(2), 742–864.