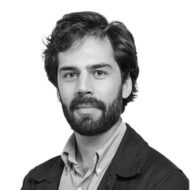
Chris J. Maddison
About
Appointed Canada CIFAR AI Chair – 2020
Chris Maddison is a Canada CIFAR AI Chair at the Vector Institute, an assistant professor at the department of computer science and statistical sciences at the University of Toronto and a research scientist at DeepMind.
Maddison develops machine learning algorithms with the long-term goal of advancing applications in the natural sciences. His focus is on improving algorithmic tools for problems like neural network training, gradient estimation, and stochastic simulation. He also enjoys collaborating on large-scale applied projects.
Awards
- Open Philanthropy AI Fellow, 2018
- IJCAI Marvin Minsky Medal for Outstanding Achievements in AI (AlphaGo Team, 2018)
- Cannes Lion International Festival of Creativity, Grand Prix (AlphaGo Team, 2016)
- Google DeepMind Scholarship, 2016
- Best Paper Award, Neural Information and Processing Systems (NIPS), 2014
Relevant Publications
- Johnson, D., El Hanchi, A., Maddison, C. (2023). Contrastive Learning Can Find An Optimal Basis For Approximately View-Invariant Functions. ICLR.
- Maddison, C., Paulin, D., Teh, Y.W., Doucet, A. (2021) Dual Space Preconditioning for Gradient Descent. SIAM Journal on Optimization.
- Paulus, M., Choi, D., Tarlow, D., Krause, A., Maddison, C. (2020). Gradient Estimation with Stochastic Softmax Tricks. In Neural Information Processing Systems.
Silver, D., Huang, A., Maddison, C. J., Guez, A., Sifre, L., Van Den Driessche, G., … & Hassabis, D. (2016). Mastering the game of Go with deep neural networks and tree search. nature, 529(7587), 484-489.
Maddison, C. J., Mnih, A., & Teh, Y. W. (2016). The concrete distribution: A continuous relaxation of discrete random variables.