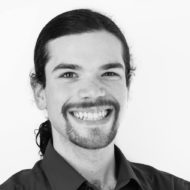
David Rolnick
About
Appointed Canada CIFAR AI Chair – 2020
David Rolnick is a Canada CIFAR AI Chair at Mila and an assistant professor in the School of Computer Science at McGill University. He is the co-founder and chair of Climate Change AI, Scientific Co-director of Sustainability in the Digital Age, and Canada lead for the NSF-NSERC Global Center on AI and Biodiversity Change (ABC).
Rolnick studies machine learning innovations for tackling climate change, as well as the mathematical foundations of deep learning algorithms. His work bridges the gap between machine learning research and fields such as energy and ecology via high-impact applications in climate change mitigation and adaptation. This work has significantly influenced international policy and entrepreneurship.
Rolnick’s work on the foundations of deep learning includes analysis of when and why different algorithms succeed or fail, as well as the development of new algorithms leveraging insights from mathematics and computational neuroscience. This work aims to build the groundwork for next-generation deep learning research by replacing trial and error progress with algorithms designed from first principles.
Awards
- AI2050 Early Career Fellow, Schmidt Sciences, 2024
- MIT Technology Review, “35 Innovators Under 35” Global List, 2021
- NSF Mathematical Sciences Postdoctoral Research Fellow, 2018-2020
- Hugh Hampton Young Memorial Fund Fellowship, 2017-2018
- NSF Graduate Research Fellowship, 2013-2017
- US-Germany Fulbright Study/ Research Scholarship, 2012-2013
Relevant Publications
- Luccioni, A.S., & Rolnick, D. (2023). Bugs in the data: How ImageNet misrepresents biodiversity. AAAI Conference on Artificial Intelligence.
Rolnick, D., Donti, P. L., Kaack, L. H., Kochanski, K., Lacoste, A., Sankaran, K., Bengio, Y. & et. al. (2022). Tackling climate change with machine learning. ACM Computing Surveys, 55(2), 1-96).
- Kaack, L.H., Donti, P.L., Strubell, E., Kamiya, G., Creutzig, F., & Rolnick, D. (2022). Aligning artificial intelligence with climate change mitigation. Nature Climate Change, 12(6), 518-527.
- Donti, P.L., Rolnick, D., & Kolter, J.Z. (2021). DC3: A learning method for optimization with hard constraints. International Conference on Learning Representations.
Rolnick, D., Ahuja, A., Schwarz, J., Lillicrap, T., & Wayne, G. (2019). Experience replay for continual learning. In Advances in Neural Information Processing Systems (pp. 350-360).
Hanin, B., & Rolnick, D. (2019). Complexity of linear regions in deep networks.