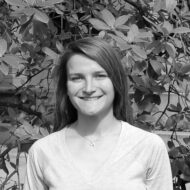
Elina Robeva
Appointment
Canada CIFAR AI Chair
Pan-Canadian AI Strategy
About
Appointed Canada CIFAR AI Chair – 2024
Robeva’s research lies at the intersection of mathematical statistics, machine learning, combinatorics, multilinear algebra, algebraic statistics, and applied algebraic geometry.
She develops machine learning and optimization methods for inference in models that depict complex dependencies in data. She addresses situations in which many commonly made yet unrealistic assumptions do not hold by leveraging the mathematical structure of the model at hand.
Robeva’s work spans causal inference, graphical models, tensor decomposition, non-parametric density estimation, hidden variable models, and super-resolution imaging. More precisely, she develops theory and algorithms for:
- causal inference for observational data (both temporal and non-temporal) in the presence of hidden variables and causal feedback loops;
- tensor decomposition applied to machine learning problems;
- sparse inverse problems, such as super-resolution imaging;
- high-dimensional, non-parametric density estimation leveraging dependencies between the variables.
Awards
- André Aisenstadt Prize, Center de Recherches Mathématiques (2023)
- CAIMS/PIMS Early Career Research Award, CAIMS (2022)
- UBC/PIMS Mathematical Sciences Young Faculty Award, UBC (2020)
- SIAM Algebraic Geometry Early Career Prize, SIAM (2019)
- Bernard Friedman Memorial Prize in Applied Mathematics, UC Berkeley (2016)
Relevant Publications
- Robeva, E. (2016). “Orthogonal Decomposition of Symmetric Tensors.” SIAM Journal on Matrix Analysis and Applications, 37, pp. 86—102.
- Liu, Y., Robeva, E., & Wang, H. (2021). “Learning Linear Non-Gaussian Graphical Models with Multidirected Edges.” Journal of Causal Inference, 9.1, pp. 250—263.
- Kubjas. K, Robeva, E., & Sturmfels, B. (2015). “Fixed Points of the EM Algorithm and Nonnegative Rank Boundaries.” Annals of Statistics, 43.1, pp. 422—461.
- Robeva. E, & Seigal, A. (2019). “Duality of Graphical Models and Tensor Networks.” Information and Inference, 8.1, pp. 273—288.
- Kurmanbek, B., & Robeva, E. (2023). “Multivariate Super-Resolution without Separation.” Information and Inference, 12.3, pp. 1938—1963.