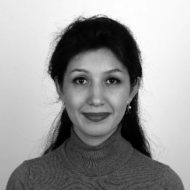
Golnoosh Farnadi
Appointment
Canada CIFAR AI Chair
National Program Committee member
Pan-Canadian AI Strategy
Connect
About
Appointed Canada CIFAR AI Chair – 2021
Golnoosh Farnadi is a Canada CIFAR AI Chair at Mila and an assistant professor at Université de Montréal.
The increasing use of algorithmic decision making in domains that affect people’s lives such as employment, education, policing and loan approval, has raised concerns about possible biases and discrimination that such systems might introduce. Recent concerns about algorithmic discrimination have motivated the development of fairness-aware mechanisms in the machine learning (ML) community and the operations research (OR) community, independently. While in fairness-aware ML, the focus is usually on ensuring that the predictions made by a learned model are fair, in reality the fairness should be guaranteed for the decisions made using such predictions. Existing methods in fairness-aware optimization resolve this issue, however they are often deterministic and fall short in exploiting the knowledge which is available in data.
Farnadi’s research focuses on the complementary strengths of fairness methods in ML and OR to address these shortcomings in a fair data-driven decision making system.
Awards
- Postdoctoral fellow scholarship, IVADO, 2018 - 2021
- Best paper award, Beyond online data workshop at ICWSM, 2018
- Best student paper award, ILP, 2015
Relevant Publications
Sivaraman, A., Farnadi, G., Millstein, T., & Van den Broeck, G. (2020). Counterexample-Guided Learning of Monotonic Neural Networks. Advances in Neural Information Processing Systems, 33.
Farnadi, G., Babaki, B., & Gendreau, M. (2020). A Unifying Framework for Fairness-Aware Influence Maximization. In Companion Proceedings of the Web Conference 2020 (pp. 714-722).
Choi, Y., Farnadi, G., Babaki, B., & Van den Broeck, G. (2020). Learning fair naive bayes classifiers by discovering and eliminating discrimination patterns. In Proceedings of the AAAI Conference on Artificial Intelligence (Vol. 34, No. 06, pp. 10077-10084).
Farnadi, G., Babaki, B., & Getoor, L. (2018). Fairness in relational domains. In Proceedings of the 2018 AAAI/ACM Conference on AI, Ethics, and Society (pp. 108-114).
Support Us
CIFAR is a registered charitable organization supported by the governments of Canada and Quebec, as well as foundations, individuals, corporations and Canadian and international partner organizations.