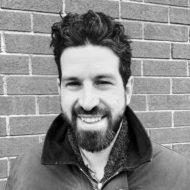
Guillaume Lajoie
Appointment
Canada CIFAR AI Chair
Pan-Canadian AI Strategy
About
Appointed Canada CIFAR AI Chair – 2021
Guillaume Lajoie is a Canada CIFAR AI Chair at Mila and an associate professor in the department of mathematics and statistics at Université de Montréal. He also holds a Canada Research Chair in Neural Computations and Interfacing.
Lajoie’s research is positioned at the intersection of AI and Neuroscience where he develops tools to better understand mechanisms of intelligence common to both biological and artificial systems. His research group’s contributions range from advances in multi-scale learning paradigms for large artificial systems, to applications in neurotechnology. Lajoie is actively involved in responsible AI development efforts, seeking to identify guidelines and best practices for use of AI in research and beyond.
Awards
- Simons Collaborations on the Global Brain Pilot Award, 2021
- Google Research Faculty Award, 2020
- Research Scholar award from the Fonds de Recherche du Québec en Santé (FRQS), 2018
- Washington Research Foundation Innovation Fellow, 2015
- Bernstein Fellow in Computational Neuroscience, 2014
Relevant Publications
- Mao, X., Chang, Y-C., Zanos, S., & Lajoie, G. (2024). Personalized inference for neurostimulation with meta-learning: a case study of vagus nerve stimulation. Journal of Neural Engineering, 21(2).
- Bredenberg, C., Williams, E., Savin, C., Richards, B., & Lajoie, G. (2023). Formalizing locality for normative synaptic plasticity models. NeurIPS.
- Mittal, S., Bengio, Y., Lajoie, G. (2022). Is a Modular Architecture Enough? NeurIPS.
Pezeshki, M., Kaba, S. O., Bengio, Y., Courville, A., Precup, D., & Lajoie, G. (2021). Gradient Starvation: A Learning Proclivity in Neural Networks. arXiv preprint arXiv:2011.09468.
Kerg, G., Kanuparthi, B., Goyal, A., Goyette, K., Bengio, Y., & Lajoie, G. (2020). Attention: untangling tradeoffs in self-attentive neural networks, Second Symposium on Machine Learning and Dynamical Systems. arXiv preprint arXiv:2006.09471.
Laferrière, S., Bonizzato, M., Côté, S. L., Dancause, N., & Lajoie, G. (2020). Hierarchical Bayesian optimization of spatiotemporal neurostimulations for targeted motor outputs. IEEE Transactions on Neural Systems and Rehabilitation Engineering.
Kerg, G., Goyette, K., Touzel, M. P., Gidel, G., Vorontsov, E., Bengio, Y., & Lajoie, G. (2019). Non-normal Recurrent Neural Network (nnRNN): learning long time dependencies while improving expressivity with transient dynamics. In Advances in Neural Information Processing Systems (pp. 13613-13623).