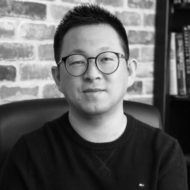
Lei Ma
Appointment
Canada CIFAR AI Chair
Pan-Canadian AI Strategy
About
Appointed Canada CIFAR AI Chair – 2021
Lei Ma is a Canada CIFAR AI Chair and a fellow at Amii. He is an associate professor in the Department of Electrical & Computer Engineering at the University of Alberta.
Ma’s research focuses on providing both fundamental quality assurance methodology and systematic engineering support for building complex AI systems to make them more reliable, safe and secure, bridging the gap between AI and its real-world applications. He is continuously proposing novel techniques of quality assessment, quality issue detection, localization, root cause analysis, repairing, etc., specially designed for both AI models at the unit level and AI systems at the whole system level, to address the AI quality concerns across domains, e.g., autonomous driving, video games, DeepFake,and healthcare.
Keywords
Machine learning; artificial intelligence; explainable AI; system engineering; quality, reliability, safety and security in machine learning; testing, debugging, repairing of machine learning systems; applied machine learning (autonomous driving, cyber-physical systems, etc)
Awards
- ACM SIGSOFT Distinguished Paper Award, The 34th IEEE/ACM Conference on Automated Software Engineering (ASE 2019), Nov. 2019
- Baidu-NASAC Academic Star Award, China Computer Federation Technical Committee on Software Engineering (CCF TCSE), Nov. 2018
- ACM SIGSOFT Distinguished Paper Award, The 33rd IEEE/ACM International Conference on Automated Software Engineering (ASE 2018), Sep. 2018
- ACM SIGSOFT Distinguished Paper Award, The 30th IEEE/ACM International Conference on Automated Software Engineering (ASE 2015), Nov. 2015
- Championship & Best Testing Tool Award, 2015 International Search-Based Software Testing Competition, May 2015
Relevant Publications
Guo, Q., Juefei-Xu, F., Xie, X., Ma, L., Wang, J., Yu, B., … & Liu, Y. (2020). Watch out! motion is blurring the vision of your deep neural networks. Advances in Neural Information Processing Systems, 33.
Peng, Z., Yang, J., Chen, T. H., & Ma, L. (2020). A first look at the integration of machine learning models in complex autonomous driving systems: a case study on Apollo. In Proceedings of the 28th ACM Joint Meeting on European Software Engineering Conference and Symposium on the Foundations of Software Engineering (pp. 1240-1250).
Du, X., Xie, X., Li, Y., Ma, L., Liu, Y., & Zhao, J. (2019). Deepstellar: Model-based quantitative analysis of stateful deep learning systems. In Proceedings of the 2019 27th ACM Joint Meeting on European Software Engineering Conference and Symposium on the Foundations of Software Engineering (pp. 477-487).
Ma, L., Juefei-Xu, F., Zhang, F., Sun, J., Xue, M., Li, B., … & Wang, Y. (2018). Deepgauge: Multi-granularity testing criteria for deep learning systems. In Proceedings of the 33rd ACM/IEEE International Conference on Automated Software Engineering (pp. 120-131).
Ma, L., Artho, C., Zhang, C., Sato, H., Gmeiner, J., & Ramler, R. (2015). Grt: Program-analysis-guided random testing (t). In 2015 30th IEEE/ACM International Conference on Automated Software Engineering (ASE) (pp. 212-223). IEEE.
Support Us
CIFAR is a registered charitable organization supported by the governments of Canada and Quebec, as well as foundations, individuals, corporations and Canadian and international partner organizations.