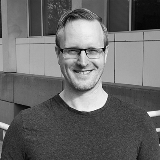
Mark Schmidt
Appointment
Canada CIFAR AI Chair
Pan-Canadian AI Strategy
About
Appointed Canada CIFAR AI Chair – 2019
Mark Schmidt is a Canada CIFAR AI Chair, a fellow at Amii, and an associate professor in the Laboratory for Computational Intelligence at the University of British Columbia. He is a Faculty Fellow with ElementAI and a consultant for 1QBit and LimeSpot.
Schmidt explores the challenges that come with learning complicated models from large datasets. His work is mainly focused on accelerating and verifying fundamental machine learning algorithms. He works in the areas of optimization for machine learning, probabilistic machine learning, computer vision applications among others.
Schmidt’s work improves the speed, efficiency and effectiveness of machine learning models. He has applied his work in computer vision toward recognizing distinct objects in images, outdoor image segmentation and depth estimation and for image restoration and inpainting. Schmidt has also developed applications to analyze the propagation of ideas in social networks, for natural language sequence labeling and for modeling the kinematics of DNA strands.
Awards
- Best Paper Award, AISTAT, 2021
- Lagrange Prize in Continuous Optimization, the Mathematical Optimization Society, 2018
- Best Student Paper Award, In International Conference on DNA-Based Computers, 2017
- Sloan Research Fellowship, 2017
- NSERC Postdoctoral Fellowship, 2012
Relevant Publications
Kunstner, F., Kumar, R., & Schmidt, M. (2021). Homeomorphic-Invariance of EM: Non-Asymptotic Convergence in KL Divergence for Exponential Families via Mirror Descent. In International Conference on Artificial Intelligence and Statistics (pp. 3295-3303).
Zolaktaf, S., Dannenberg, F., Rudelis, X., Condon, A., Schaeffer, J. M., Schmidt, M., … & Winfree, E. (2017). Inferring parameters for an elementary step model of DNA structure kinetics with locally context-dependent Arrhenius rates. In International Conference on DNA-Based Computers (pp. 172-187).
Schmidt, M., Le Roux, N., & Bach, F. (2017). Minimizing finite sums with the stochastic average gradient. Mathematical Programming, 162(1-2), 83-112.
Roux, N. L., Schmidt, M., & Bach, F. (2012). A stochastic gradient method with an exponential convergence rate for finite training sets.
Schmidt, M., Berg, E., Friedlander, M., & Murphy, K. (2009). Optimizing costly functions with simple constraints: A limited-memory projected quasi-newton algorithm. In Artificial Intelligence and Statistics (pp. 456-463).
Support Us
CIFAR is a registered charitable organization supported by the governments of Canada and Quebec, as well as foundations, individuals, corporations and Canadian and international partner organizations.