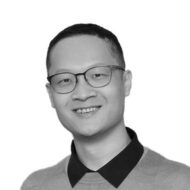
Mo Chen
Appointment
Canada CIFAR AI Chair
Pan-Canadian AI Strategy
About
Appointed Canada CIFAR AI Chair – 2020
Mo Chen is a Canada CIFAR AI Chair and a fellow at Amii. He is an assistant professor in the School of Computing Science at Simon Fraser University (SFU). He also directs the Multi-Agent Robotic Systems Lab at SFU and is a Distal Fellow of the NSERC Canadian Robotics Network.
Chen directs the Multi-Agent Robotic Systems Lab at Simon Fraser University. The lab’s research focuses on principled robotic decision making, centered around combining traditional analytical methods in robotics and modern data-driven techniques. The lab addresses theoretical and computational challenges in robotic safety and human-robot interactions. This is achieved by connecting control theory, computer vision, and reinforcement learning. Through incorporating human knowledge and understanding of robotic systems into data-driven algorithms, Chen aspires to make robots safer and smarter to enable more widespread use of robotic systems such as autonomous cars, unmanned aerial vehicles, and household robots. Chen’s interdisciplinary research spans the fields of control theory, robotic safety verification, computer vision, reinforcement learning, and human-robot interactions.
Awards
- Oral paper presentation at the Conference on Robot Learning, 2023
- Best Paper Award on Cognitive Robotics at the IEEE International Conference on Intelligent Robots and Systems, 2022
- Eli Jury Award, 2017
- Demetri Angelakos Memorial Achievement Award, 2016
Relevant Publications
- Xubo Lyu, Hanyang Hu, Seth Siriya, Ye Pu, and Mo Chen, “Task-Oriented Koopman-Based Control with Contrastive Encoder,” in Conference on Robot Learning, 2023.
- Payam J. Yazdian, Mo Chen, and Angelica Lim, “Gesture2vec: Clustering gestures using representation learning methods for co-speech gesture generation,” in International Conference on Intelligent Robots and Systems, Best Paper Award on Cognitive Robotics, 2022.
- Minh Bui, Michael Lu, Reza Hojabr, Mo Chen, and Arrvindh Shriraman, “Real-Time Formal Verification of Autonomous Systems With An FPGA,” in International Conference on Intelligent Robots and Systems, 2021.
- Payam Nikdel, Richard Vaughan, and Mo Chen, “LBGP: Learning Based Goal Planning for Autonomous Following in Front,” in International Conference on Robotics and Automation, 2021.
- Mo Chen, Sylvia L. Herbert, Haimin Hu, Ye Pu, Jaime F. Fisac, Somil Bansal, SooJean Han, and Claire J. Tomlin, “FaSTrack: a Modular Framework for Real-Time Motion Planning and Guaranteed Safe Tracking,” IEEE Transactions on Automatic Control, vol. 66, pp. 5861–5876, Dec. 2021.
- Anjian Li, Somil Bansal, George Giovanis, Varun Tolani, Claire J. Tomlin, and Mo Chen, “Generating Robust Supervision for Learning-Based Visual Navigation Using Hamilton-Jacobi Reachability,” in Conference on Learning for Dynamics and Control, 2020.