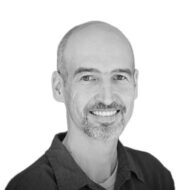
Nathan Sturtevant
Appointment
Canada CIFAR AI Chair
Pan-Canadian AI Strategy
About
Appointed Canada CIFAR AI Chair – 2020
Nathan Sturtevant is a Canada CIFAR AI Chair, former Director and current fellow at Amii, and a professor in the Department of Computing Science at the University of Alberta, where he leads the MovingAI Lab.
Sturtevant’s research focuses on heuristic and combinatorial search problems, including both theoretical and applied approaches. Particular applications for his research include pathfinding and planning in memory-constrained real-time environments (e.g. commercial video games) as well as algorithms for building and using memory-based heuristics via large-scale search. His research has been implemented in commercial video games and he continues to collaborate with practitioners in the games industry.
Awards
- Service Award, Symposium on Combinatorial Search 2024
- Prominent Paper Award, AIJ, 2021
- ICAPS System Demonstration Silver Medal, 2021
- Best Paper Award, Symposium on Combinatorial Search, 2018, 2019
- Senior Member, Association for the Advancement of Artificial Intelligence, 2017
- Outstanding Paper Award, Association for the Advancement of Artificial Intelligence (AAAI), 2016
Relevant Publications
- Mashayekhi, R., Atzmon, D., & Sturtevant, N. R. (2023). Analyzing and improving the use of the FastMap embedding in pathfinding tasks. Proceedings of the AAAI Conference on Artificial Intelligence, 37(10), 12473-12481.
- Chen, J., & Sturtevant, N. R. (2021). Necessary and sufficient conditions for avoiding reopenings in best first suboptimal search with general bounding functions. Proceedings of the AAAI Conference on Artificial Intelligence, 35(5), 3688-3696.
- Atzmon, D., Stern, R., Felner, A., Sturtevant, N. R., & Koenig, S. (2020). Probabilistic robust multi-agent path finding. Proceedings of the International Conference on Automated Planning and Scheduling, 30, 29-37.
Stern, R., Sturtevant, N. R., Felner, A., Koenig, S., Ma, H., Walker, T. T., … & Boyarski, E. (2019). Multi-agent pathfinding: Definitions, variants, and benchmarks. In Twelfth Annual Symposium on Combinatorial Search.
Sharon, G., Stern, R., Felner, A., & Sturtevant, N. R. (2015). Conflict-based search for optimal multi-agent pathfinding. Artificial Intelligence, 219, 40-66.