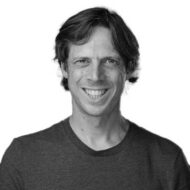
Nicolas Le Roux
About
Appointed Canada CIFAR AI Chair – 2020
Nicolas Le Roux is a Canada CIFAR AI Chair at Mila and an adjunct professor at McGill University’s School of Computer Science and Université de Montréal’s Department of Computer Science and Operations Research. He is a Research Scientist at Microsoft Research in Montréal.
Le Roux is interested in understanding how the interplay between noise and curvature affects convergence speed and generalization in stochastic optimization and understanding the dynamics of optimization in reinforcement learning, especially policy-gradient methods.
Awards
- Best Paper Award, Runner up, AISTATS, 2022
- Co-Recipient of the Lagrange Prize in Continuous Optimization, Society for Industrial and Applied Mathematics (SIAM), 2018
Relevant Publications
- Sordoni, A., Yuan, X., Côté, M-A., Pereira, M., Trischler, A., Xiao, Z., Hosseini, A., Niedtner, F., & Le Roux, N. (2023). Joint Prompt Optimization of Stacked LLMs using Variational Inference. Thirty-seventh Conference on Neural Information Processing Systems.
- Vaswani, S., Bachem, O., Totaro, S., Müller, R., Garg, S., Geist, M., Machado, M.C., Castro, P.S., & Le Roux, N. (2022). A general class of surrogate functions for stable and efficient reinforcement learning. AISTATS (pp. 8619-8649).
- Thomas, V., Pedregosa, F., Merriënboer, B., Manzagol, P.A., Bengio, Y. & Le Roux, N. (2020). On the interplay between noise and curvature and its effect on optimization and generalization. International Conference on Artificial Intelligence and Statistics
- Z Ahmed, N Le Roux, M Norouzi, D Schuurmans (2019). Understanding the impact of entropy on policy optimization. International Conference on Machine Learning, 151-160
Le Roux, N., & Bengio, Y. (2008). Representational power of restricted Boltzmann machines and deep belief networks. Neural computation, 20(6), 1631-1649.
Bengio, Y., Paiement, J. F., Vincent, P., Delalleau, O., Roux, N., & Ouimet, M. (2003). Out-of-sample extensions for lle, isomap, mds, eigenmaps, and spectral clustering. Advances in neural information processing systems, 16, 177-184.