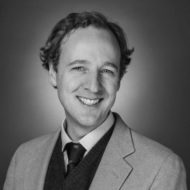
O. Anatole von Lilienfeld
Appointment
Canada CIFAR AI Chair
Pan-Canadian AI Strategy
About
Appointed Canada CIFAR AI Chair – 2022
Anatole von Lilienfeld is a Canada CIFAR AI Chair at the Vector Institute. He leads an interdisciplinary team at University of Toronto, working on theoretical and computational methods for quantum mechanics and statistical mechanics based exploration of chemical compound space. While his research falls into the realm of physical chemistry, it relies on theory and computations as well as methods based in physics, mathematics, and computer sciences. Rather than conducting primary experiments, von Lilienfeld’s team compares computational predictions to experimental results reported in the scientific literature, or via direct collaborations with experimentalists.
Von Lilienfeld’s work deals with chemical compound space, relying on conceptual as well as molecular grand-canonical ensemble density functional theory, ligand design and intermolecular binding, quantum chemistry (solving approximate Schroedinger equations with density functional theory, semi-empirical methods, or post-Hartree Fock), machine learning (supervised and unsupervised learning), statistical mechanics (using electronic, atomistic, coarse-grained & empirical force-fields and multi-scaling), molecules, liquids, and solids including molecular crystals and defects, and supercomputing.
Awards
- Löwdin Lecturer Award, Uppsala University, 2021
- Feynman Prize Theory, Foresight Institute, 2018
- Woodward Lecturer, Harvard Chemistry, 2014
- Thomas Kuhn Paradigm Shift Award, OpenEye, 2013
- Award for excellence, LDRD Sandia Laboratories, 2010
Relevant Publications
- Back, S., Aspuru-Guzik, A., Ceriotti, M., Gryn'ova, G., Grzybowski, B., Gu, G. H., Hein, J., Hippalgaonkar, K., Hormázabal, R., Jung, Y., Kim, S., Kim, W. Y., Moosavi, S. M., Noh, J., Park, C., Schrier, J., Schwaller, P., Tsuda, K., Vegge, T., von Lilienfeld, O. A., & Walsh, A. (2024). Accelerated chemical science with AI. Digital Discovery, 3(1), 23-33. Royal Society of Chemistry.
- Huang, B., von Rudorff, G. F., & von Lilienfeld, O. A. (2023). The central role of density functional theory in the AI age. Science, 381(6654), 170-175.
- Karandashev, K., & von Lilienfeld, O. A. (2022). An orbital-based representation for accurate quantum machine learning. The Journal of Chemical Physics, 156(11).
- D Lemm, D., GF von Rudorff, OA von Lilienfeld. (2021). Machine learning based energy-free structure predictions of molecules, transition states, and solids. Nature Communications, 12.
- B Huang, OA von Lilienfeld. (2020). Quantum machine learning using atom-in-molecule-based fragments selected on the fly. Nature Chemistry, 12.
- M Rupp, A Tkatchenko, KR Müller, OA von Lilienfeld. (2012). Fast and accurate modeling of molecular atomization energies with machine learning. Physical Review Letters.