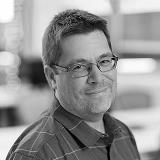
Pascal Vincent
Appointment
Associate Fellow
Learning in Machines & Brains
About
Pascal Vincent is an Associate Fellow in CIFAR’s Learning in Machines & Brains program and a Canada CIFAR AI Chair at Mila. He is an associate professor at the Department of Computer Science and Operations Research (DIRO) at Université de Montréal.
Vincent’s current research interests in the statistical machine learning field include unsupervised feature learning, manifold modelling, alternative parameter estimation techniques for energy based models, semi-supervised learning and learning of deep neural-network architectures. His current main focus is on fundamental principles and techniques for extracting meaningful high level distributed representations from raw high dimensional sensory inputs. Vincent’s work on regularizing auto-encoders (denoising and contractive variants) for unsupervised feature and deep network learning was at the heart of the strategy that won the 2011 Unsupervised and Transfer Learning Challenge.
Awards
- Best Student Paper Award, ICML, 2012
- Outstanding Student Paper (Honorable Mention), NIPS, 2011
Relevant Publications
Bengio, Y., Courville, A., & Vincent, P. (2013). Representation learning: A review and new perspectives. IEEE transactions on pattern analysis and machine intelligence, 35(8), 1798-1828.
Vincent, P., Larochelle, H., Lajoie, I., Bengio, Y., Manzagol, P. A., & Bottou, L. (2010). Stacked denoising autoencoders: Learning useful representations in a deep network with a local denoising criterion. Journal of machine learning research, 11(12).
Erhan, D., Courville, A., Bengio, Y., & Vincent, P. (2010). Why does unsupervised pre-training help deep learning?. In Proceedings of the thirteenth international conference on artificial intelligence and statistics (pp. 201-208). JMLR Workshop and Conference Proceedings.
Vincent, P., Larochelle, H., Bengio, Y., & Manzagol, P. A. (2008). Extracting and composing robust features with denoising autoencoders. In Proceedings of the 25th international conference on Machine learning (pp. 1096-1103).
Bengio, Y., Ducharme, R., Vincent, P., & Janvin, C. (2003). A neural probabilistic language model. The journal of machine learning research, 3, 1137-1155.
Support Us
CIFAR is a registered charitable organization supported by the governments of Canada and Quebec, as well as foundations, individuals, corporations and Canadian and international partner organizations.