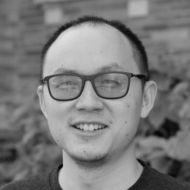
Renjie Liao
About
Appointed Canada CIFAR AI Chair – 2023
Renjie Liao’s research focuses on geometric deep learning, deep generative models, and probabilistic inference. He has developed neural networks that can learn useful graph representations and can generate high-quality graph-structured data (e.g., molecules and proteins). His research contributions also include developing theoretical understandings of such models and applying them to solve practical problems in computer vision, self-driving, and reinforcement learning. Renjie’s long-term research goal is to build learning systems that can automatically discover structures from data and reason over structures.
Awards
- Top Reviewer, ICML (2020)
- Best Reviewer, NeurIPS (2019)
- Best Paper Award, ICML Workshop on Tractable Probabilistic Modeling (2019)
- RBC Graduate Fellowship, RBC (2019-2021)
- Connaught International Scholarship for Doctoral Students, University of Toronto (2015-2019)
Relevant Publications
- Liao, R., Kornblith, S., Ren, M., Fleet, D. J., & Hinton, G. (2022). Gaussian-Bernoulli RBMs Without Tears. arXiv preprint arXiv:2210.10318.
- Liao, R., Urtasun, R., & Zemel, R. (2021). A PAC-Bayesian Approach to Generalization Bounds for Graph Neural Networks. In International Conference on Learning Representations (ICLR).
- Liao, R., Li, Y., Song, Y., Wang, S., Hamilton, W., Duvenaud, D. K., ... & Zemel, R. (2019). Efficient graph generation with graph recurrent attention networks. Advances in neural information processing systems (NeurIPS).
- Liao, R., Zhao, Z., Urtasun, R., & Zemel, R. (2019). LanczosNet: Multi-Scale Deep Graph Convolutional Networks. In International Conference on Learning Representations (ICLR).
- Liao, R., Xiong, Y., Fetaya, E., Zhang, L., Yoon, K., Pitkow, X., ... & Zemel, R. (2018). Reviving and improving recurrent back-propagation. In International Conference on Machine Learning (ICML). Profile Link (two max) – e.g. your own webpage, Research Gate, Google Scholar: Homepage: https://lrjconan.github.io/