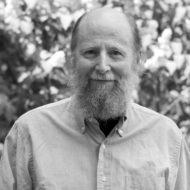
Richard S. Sutton
Appointment
Associate Fellow
Canada CIFAR AI Chair
Learning in Machines & Brains
Pan-Canadian AI Strategy
About
Appointed Canada CIFAR AI Chair – 2021
Richard S. Sutton is a Canada CIFAR AI Chair and a Distinguished Fellow of CIFAR’s Learning in Machines & Brains program. He is the Chief Scientific Advisor of Amii, a Distinguished Research Scientist at DeepMind and a Professor at the University of Alberta’s Department of Computing Science.
Sutton is one of the pioneers of reinforcement learning, an approach to artificial and natural intelligence that emphasizes learning and planning from sample experience, and a field in which he continues to lead the world. He is most interested in understanding what it means to be intelligent, to predict and influence the world, to learn, perceive, act, and think. He seeks to identify general computational principles underlying what we mean by intelligence and goal-directed behaviour. Over his career, he has made a number of significant contributions to the field, including the theory of temporal-difference learning, the actor-critic (policy gradient) class of algorithms, the Dyna architecture (integrating learning, planning and reacting), the Horde architecture, and gradient and emphatic temporal-difference algorithms. Sutton seeks to extend reinforcement learning ideas to an empirically grounded approach to knowledge representation based on prediction.
Awards
- Senior Fellow, CIFAR program in Learning in Machines and Brains, 2018-present
- Lifetime Achievement Award and Fellow of the Canadian Artificial Association (CAIAC), 2018
- Fellow, Royal Society of Canada, 2017
- Fellow, Association for the Advancement of Artificial Intelligence, 2007-2010
Relevant Publications
Sutton, R.S., and A.G. Barto. Reinforcement Learning: An Introduction. Cambridge, MA: MIT Press, Second Edition, 2018.
Sutton, R.S., McAllester, D., Singh, S., Mansour, Y. (2000). Policy Gradient Methods for Reinforcement Learning with Function Approximation. Advances in Neural Information Processing Systems 12 (Proceedings of the 1999 conference), pp. 1057-1063. MIT Press.
Sutton, R.S., Precup, D., Singh, S. (1999). Between MDPs and semi-MDPs: A Framework for Temporal Abstraction in Reinforcement Learning. Artificial Intelligence 112:181-211.
Sutton, R.S., Barto, A.G. (1990). Time-derivative models of pavlovian reinforcement. In Learning and Computational Neuroscience: Foundations of Adaptive Networks, M. Gabriel and J. Moore, Eds., pp. 497-537. MIT Press.
Sutton, R. S. (1988). Learning to predict by the methods of temporal differences. Machine Learning 3: 9-44.
Support Us
CIFAR is a registered charitable organization supported by the governments of Canada and Quebec, as well as foundations, individuals, corporations and Canadian and international partner organizations.