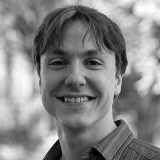
Simon Lacoste-Julien
Appointment
Associate Fellow
Canada CIFAR AI Chair
Learning in Machines & Brains
Pan-Canadian AI Strategy
About
Appointed Canada CIFAR AI Chair – 2018
Simon Lacoste-Julien is an associate fellow in the CIFAR Learning in Machines & Brains program and Canada CIFAR AI Chair at Mila. He is an associate professor at the Department of Computer Science and Operations Research (DIRO) at Université de Montréal and is the part-time VP Lab Director at the Samsung SAIT AI Lab in Montreal.
Simon Lacoste-Julien’s research focuses on machine learning, i.e., how to program a computer to learn from data and solve useful tasks. His primary research goal is to develop and analyze machine learning techniques that can exploit, at large scale, the rich structure of data in interdisciplinary applications such as natural language processing, information retrieval, computer vision and computational biology. To this end, he combines tools from optimization, statistics and computer science, and particularly enjoys working at the interface between domains. Lacoste-Julien is best known for his contributions in three areas: structured prediction (classification problems where the outputs are structured objects such as sequences or graphs); large scale optimization (incremental gradient method and Frank-Wolfe optimization); and the combination of generative and discriminative methods.
Awards
- Google Focused Research Award, 2016
- NIPS Best Reviewer Award, 2015
- Google Faculty Research Award, 2015
- Research in Paris Fellowship, City of Paris, 2011-2012
- Wolfson College Junior Research Fellowship, University of Cambridge, 2009-2011
Relevant Publications
Yaakoubi, Y., Soumis, F., & Lacoste-Julien, S. (2021). Structured Convolutional Kernel Networks for Airline Crew Scheduling.
Kwon, N., Na, H., Huang, G., & Lacoste-Julien, S. (2021). Repurposing Pretrained Models for Robust Out-of-domain Few-Shot Learning.
Larsen, E., Lachapelle, S., Bengio, Y., Frejinger, E., Lacoste-Julien, S., & Lodi, A. (2018). Predicting tactical solutions to operational planning problems under imperfect information.
Arpit, D., Jastrzębski, S., Ballas, N., Krueger, D., Bengio, E., Kanwal, M. S., … & Lacoste-Julien, S. (2017, July). A closer look at memorization in deep networks. In International Conference on Machine Learning (pp. 233-242). PMLR.
Defazio, A., Bach, F., & Lacoste-Julien, S. (2014). SAGA: A fast incremental gradient method with support for non-strongly convex composite objectives. In Advances in neural information processing systems (pp. 1646-1654).
Support Us
CIFAR is a registered charitable organization supported by the governments of Canada and Quebec, as well as foundations, individuals, corporations and Canadian and international partner organizations.