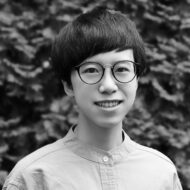
Xiaoxiao Li
About
Appointed Canada CIFAR AI Chair – 2024
Xiaoxiao Li is an Assistant Professor in the Electrical and Computer Engineering Department at the University of British Columbia (UBC), leading the Trusted and Efficient AI (TEA) Group, and an Adjunct Assistant Professor at the School of Medicine at Yale University. Li specializes in the interdisciplinary field of deep learning and biomedical data analysis. Her primary mission is to make AI more reliable, especially when it comes to sensitive areas like healthcare.
At the TEA Group, her team explores a wide range of topics from fundamental machine learning to more focused healthcare-driven AI solutions. The group delves into topics like learning from multimodal and heterogeneous data, efficient AI models, federated learning, and creating AI models that not only perform tasks but can also be trustworthy. Groundbreaking work from the team includes AI-driven analysis of diverse data including neuroimages, pathology slides, as well as molecular and clinical notes.
In essence, Li’s work is all about bridging the world of advanced machine learning with the practical needs of the healthcare field.
Awards
- ICLR Outstanding Reviewer Award (2023)
- Meta Research Award (2022)
- Nvidia Hardware Award (2021)
- MICCAI Student Travel Award (2020)
- Yale Advanced Graduate Leadership Fellowship (2018-2020)
Relevant Publications
- Li, X., Jiang, M., Zhang, X., Kamp, M., & Dou, Q. (2021). Fedbn: Federated learning on non-iid features via local batch normalization. ICLR 2021.
- Li, X., Zhou, Y., Dvornek, N., Zhang, M., Gao, S., Zhuang, J., ... & Duncan, J. S. (2021). BrainGNN: Interpretable brain graph neural network for fmri analysis. Medical Image Analysis, 74, 102233.
- Li, X., Song, Z., & Yang, J. (2023). Federated adversarial learning: A framework with convergence analysis. ICML 2023
- Xing, S., Shen, S., Xu, B., Li, X., & Huan, T. (2023). BUDDY: molecular formula discovery via bottom-up MS/MS interrogation. Nature Methods, 1-10.
- Chen, M., Jiang, M., Dou, Q., Wang, Z., & Li, X. (2023). FedSoup: Improving Generalization and Personalization in Federated Learning via Selective Model Interpolation. MICCAI 2023