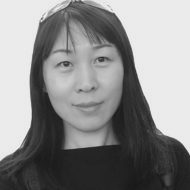
Yuhong Guo
Appointment
Canada CIFAR AI Chair
National Program Committee member
Pan-Canadian AI Strategy
About
Appointed Canada CIFAR AI Chair – 2021
Yuhong Guo is a Canada CIFAR AI Chair at Amii, a full professor in the School of Computer Science at Carleton University, and a Canada Research Chair in Machine Learning.
Guo’s research focuses on enhancing the robustness, generalizability, and practical utility of machine learning methods. She pursues a multifaceted approach, investigating key areas such as generalized transfer learning, learning under limited supervision constraints, and navigating the challenges posed by noisy or weak supervision signals. This research has produced techniques that alleviate the burden of extensive data annotation by leveraging auxiliary resources and achieving effective learning from limited or weak supervision paradigms. Guo’s research program has broadened the scope of effective machine learning to diverse scenarios and real-world challenges.
Awards
- Canada Research Chair in Machine Learning, NSERC, 2022
- Outstanding Reviewer Award, NeurIPS, 2021
- Outstanding Paper Award, AAAI, 2012
- Postdoctoral Fellowship, NSERC, 2008
- Distinguished Paper Award, IJCAI, 2005
Relevant Publications
- Tshtiak, T., En, Q., & Guo, Y. (2023). Exemplar-FreeSOLO: Enhancing Unsupervised Instance Segmentation with Exemplars. In Proceedings of the IEEE Conference on Computer Vision and Pattern Recognition (CVPR).
- Cui, W., & Guo, Y. (2021). Parameterless Transductive Feature Re-representation for Few-Shot Learning. In Proceedings of the International Conference on Machine Learning (ICML).
- Yan, Y., & Guo, Y. (2021). Adversarial Partial Multi-label Learning with Label Disambiguation. In Proceedings of the AAAI Conference on Artificial Intelligence (AAAI).
Ye, M., & Guo, Y. (2019). Progressive ensemble networks for zero-shot recognition. In Proceedings of the IEEE Conference on Computer Vision and Pattern Recognition (pp. 11728-11736).
- Ye, M., & Guo, Y. (2017). Zero-shot Classification with Discriminative Semantic Representation Learning. In Proceedings of the IEEE Conference on Computer Vision and Pattern Recognition (CVPR).
Guo, Y. (2017). Convex co-embedding for matrix completion with predictive side information. In Proceedings of the AAAI Conference on Artificial Intelligence (Vol. 31, No. 1).