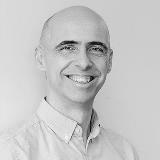
Csaba Szepesvari
Appointment
Canada CIFAR AI Chair
Pan-Canadian AI Strategy
About
Appointed Canada CIFAR AI Chair – 2020
Renewed Canada CIFAR AI Chair – 2025
Csaba Szepesvári is a Canada CIFAR AI Chair at Amii and a professor in the Computing Science Department of the University of Alberta.
Szepesvári works on reinforcement learning theory, creating and analyzing algorithms that learn efficiently and effectively while interacting with their environments in a sequential manner. He is interested in problems when a machine continuously interacts with its environment while trying to discover autonomously a good way of interacting with it. These interactive online learning problems are studied in various disciplines, such as within control theory under the name “dual control”, or within machine learning itself in the area of reinforcement learning.
Awards
- Fellow, AAAI, 2023
- Fellow, European Laboratory for Learning and Intelligent Systems, 2019
- Test of Time Award, ECML/PKDD, 2016
- UAI Best Paper Runner-Up Award, 2014
- ICML Excellent Paper Award, 2014
- Inspirational Instructor Award, Interdepartmental Science Students’ Society of the University of Alberta, 2012
Relevant Publications
- Liu, Q., Netrapalli, P., Szepesvari, C., & Jin, C. (2023). Optimistic MLE: A generic model-based algorithm for partially observable sequential decision making. In Proceedings of the 55th Annual ACM Symposium on Theory of Computing (pp. 363-376).
- Weisz, G., György, A., Kozuno, T., & Szepesvári, C. (2022). Confident Approximate Policy Iteration for Efficient Local Planning in -realizable MDPs. In Advances in Neural Information Processing Systems 35 (pp. 25547-25559).
- Zhou, D., Gu, Q., & Szepesvari, C. (2021). Nearly minimax optimal reinforcement learning for linear mixture Markov decision processes. In Conference on Learning Theory (pp. 4532-4576).
Lattimore, T., & Szepesvári, C. (2020). Bandit algorithms. Cambridge University Press.
Szepesvári, C. (2010). Algorithms for reinforcement learning. Synthesis lectures on artificial intelligence and machine learning, 4(1), 1-103.
Kocsis, L., & Szepesvári, C. (2006). Bandit based Monte-Carlo planning. In European conference on machine learning (pp. 282-293). Springer, Berlin, Heidelberg.