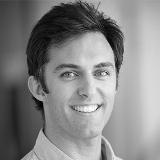
Daniel Roy
Appointment
Canada CIFAR AI Chair
Pan-Canadian AI Strategy
About
Appointed Canada CIFAR AI Chair – 2017
Renewed Canada CIFAR AI Chair – 2023
Daniel Roy is a Canada CIFAR AI Chair at the Vector Institute, an associate professor in the department of statistical sciences with a cross-appointment in the department of computer science and electrical and computer engineering at the University of Toronto. He is also an associate professor in the department of computer and mathematical sciences at the University of Toronto Scarborough.
Roy’s research blends computer science, statistics and probability theory. He studies probabilistic programming and develops computational perspectives on fundamental ideas in probability theory and statistics. He is particularly interested in representation theorems that connect computability, complexity, and probabilistic structures, stochastic processes, the use of recursion to define stochastic processes, and applications to nonparametric Bayesian statistics, and the complexity of probabilistic and statistical inference, especially in the context of probabilistic programming.
Awards
- Ontario Early Researcher Award, 2017
- Google Faculty Research Award, 2017
- Founding member, Vector Institute, 2017
- Organizer, 1st, 2nd, and 3rd NIPS Workshops on Probabilistic Programming, (2008, 2012, 2014)
- Best Poster, Conference on Bayesian Nonparametrics, 2015
Relevant Publications
Jiang, Y., Natekar, P., Sharma, M., Aithal, S. K., Kashyap, D., Subramanyam, N., … & Bengio, S. (2021). Methods and Analysis of The First Competition in Predicting Generalization of Deep Learning. In NeurIPS 2020 Competition and Demonstration Track (pp. 170-190). PMLR.
Li, M. B., Nica, M., & Roy, D. M. (2021). The Future is Log-Gaussian: ResNets and Their Infinite-Depth-and-Width Limit at Initialization.
Ramezani-Kebrya, A., Faghri, F., Markov, I., Aksenov, V., Alistarh, D., & Roy, D. M. (2021). NUQSGD: Provably Communication-efficient Data-parallel SGD via Nonuniform Quantization. Journal of Machine Learning Research, 22(114), 1-43.
Dziugaite, G. K., Hsu, K., Gharbieh, W., & Roy, D. M. (2020). On the role of data in PAC-Bayes bounds.
Jiang, Y., Foret, P., Yak, S., Roy, D. M., Mobahi, H., Dziugaite, G. K., … & Neyshabur, B. (2020). Neurips 2020 competition: Predicting generalization in deep learning.
Support Us
CIFAR is a registered charitable organization supported by the governments of Canada and Quebec, as well as foundations, individuals, corporations and Canadian and international partner organizations.