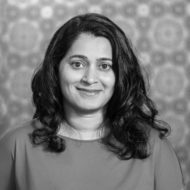
Nidhi Hegde
Appointment
Canada CIFAR AI Chair
Pan-Canadian AI Strategy
About
Appointed Canada CIFAR AI Chair – 2021
Nidhi Hegde is a Canada CIFAR AI Chair and a fellow at Amii. She is an associate professor in the Department of Computing Science at the University of Alberta.
Hegde’s research focuses on a fundamental understanding of privacy and fairness in machine learning algorithms and systems, and the design of privacy-preserving and fair algorithms. She is interested in topics such as novel optimization frameworks for fairness in machine learning, machine unlearning, and robustness of algorithms.
Relevant Publications
- Hegde, N., & Hu, B. (2022). Near-optimal thompson sampling-based algorithms for differentially private stochastic bandits. In Uncertainty in Artificial Intelligence (pp. 844-852).
- Subramanian, S.G., Poupart, P., Taylor, M.E., & Hegde, N. (2020). Multi Type Mean Field Reinforcement Learning. In International Conference on Autonomous Agents and Multi-Agent Systems
Wang, B., & Hegde, N. (2019). Privacy-preserving q-learning with functional noise in continuous spaces. In Advances in Neural Information Processing Systems (pp. 11327-11337).
Mukhopadhyay, A., Hegde, N., & Lelarge, M. (2019). Asymptotics of Replication and Matching in Large Caching Systems. IEEE/ACM Transactions on Networking, 27(4), 1657-1668.
Cecchi, F., & Hegde, N. (2017). Adaptive active hypothesis testing under limited information. In Advances in Neural Information Processing Systems (pp. 4035-4043).