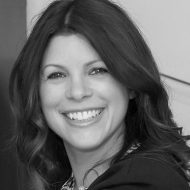
Tal Arbel
About
Appointed Canada CIFAR AI Chair – 2020
Renewed Canada CIFAR AI Chair – 2025
Tal Arbel is a Canada CIFAR AI Chair at Mila and a professor at the Department of Electrical and Computer Engineering at McGill University. She is a research director of the Probabilistic Vision Group, and Electrical & Computer Engineering “Medical Imaging Lab” at McGill University.
Arbel’s research focuses on development of probabilistic, deep learning methods in computer vision and medical image analysis, for a wide range of real-world applications, with a focus on neurological diseases. Arbel’s recent research goals are to develop new, causal models for image-based personalized medicine, where they have the potential to lead to concrete improvements in patient care. Her team is focused on the development of trustworthiness into the resulting models, including explainability, fairness and probabilistic outcomes, all of which are essential for the safety of their clinical adoption.
Relevant Publications
- A. Kumar, N. Fathi, R. Mehta, B. Nichyporuk, J. P. Falet, S. Tsaftaris, T. Arbel. (2023). Debiasing Counterfactuals In the Presence of Spurious Correlations. The 26th International Conference on Medical Image Computing and Computer Assisted Intervention (MICCAI).
- C. Shui, J. Szeto, R. Mehta, D. L. Arnold, T. Arbel. (2023). Mitigating Calibration Bias Without Fixed Attribute Grouping for Improved Fairness in Medical Imaging Analysis. The 26th International Conference on Medical Image Computing and Computer Assisted Intervention (MICCAI).
- J. Durso-Finley, J. P. Falet, R. Mehta, D. L. Arnold, N. Pawlowski, T. Arbel. (2023). Improving Image-Based Precision Medicine with Uncertainty-Aware Causal Models. The 26th International Conference on Medical Image Computing and Computer Assisted Intervention (MICCAI).
- R. Mehta, C. Shui, T. Arbel. (2023). Evaluating the Fairness of Deep Learning Uncertainty Estimates in Medical Image Analysis. The 6th Conference on Medical Imaging with Deep Learning (MIDL 2023).
- C. Shui, G. Xu, Q. Chen, J. Li, C. Ling, T. Arbel, B. Wang, C. Gagné. (2022). On Learning Fairness and Accuracy on Multiple Subgroups. The Thirty-sixth Annual Conference on Neural Information Processing Systems.
- J.D. Durso-Finley, J.P René Falet, B. Nichyporuk, D.L. Arnold and T. Arbel. (2022). Personalized Prediction of Future Lesion Activity and Treatment Effect in Multiple Sclerosis from Baseline MRI.