Reach 2024: Racing for Cause
By Kathleen Sandusky
Why our brains are so good at seeing causality (and what AI requires to catch up)
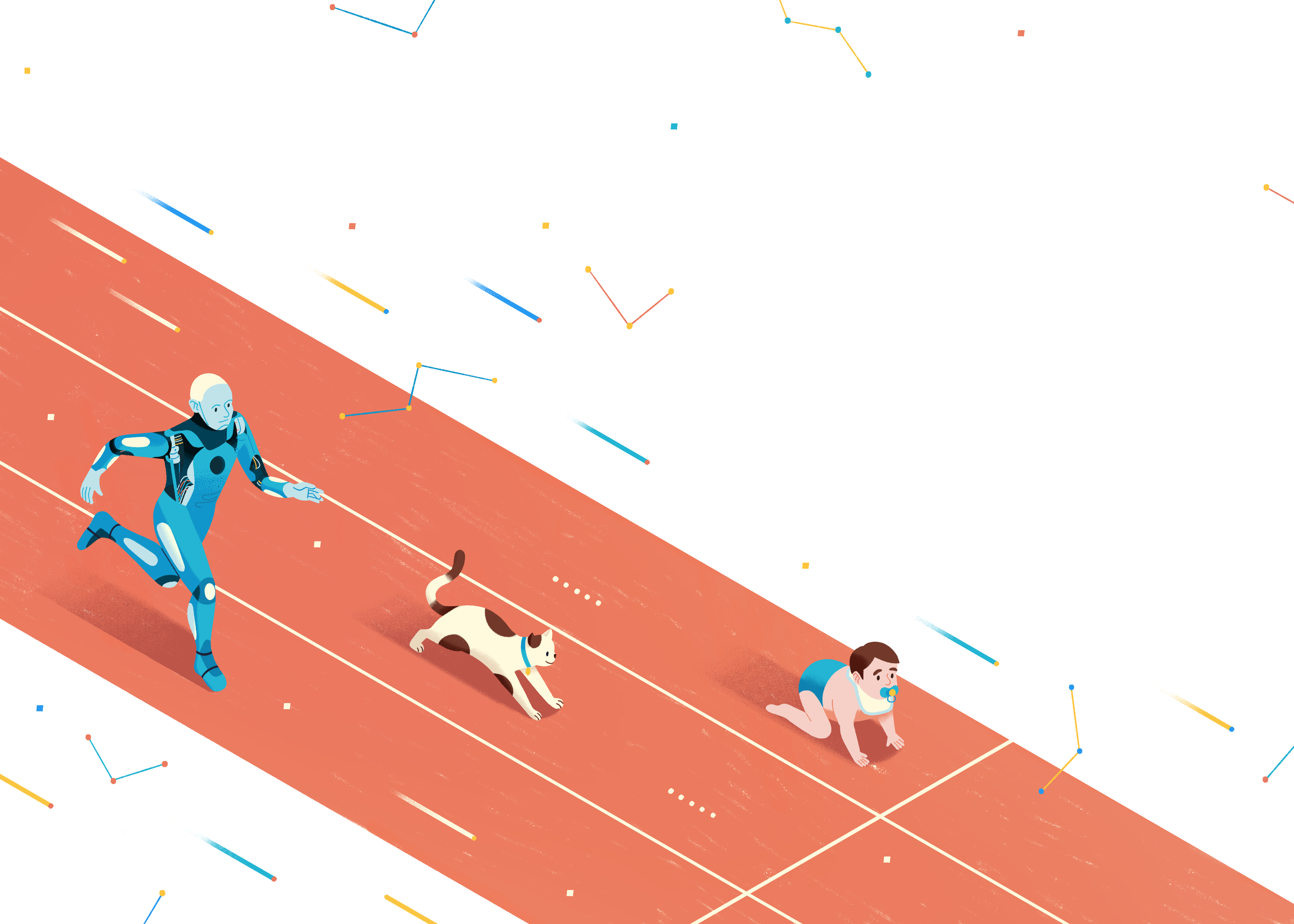
Illustration: Maya Nguyen
Babies understand it, even cats and crows seem to have some working knowledge of it. But despite huge advances, current AI systems have trouble figuring out causation: the relationship between cause and effect.
Why is the human brain so good at interpreting causation? What can neuroscience teach us about causality that can help AI and vice versa? What’s at stake?
To answer these pressing questions, we asked three CIFAR researchers for their thoughts.
ALISON GOPNIK
University of California, Berkeley Fellow, CIFAR Child & Brain Development and Learning in Machines & Brains
Alison Gopnik is a leader in studying cognitive science and children’s learning and development. In her most recent research, she combines “life history” in evolutionary biology with current research on AI to explain how children think and learn.
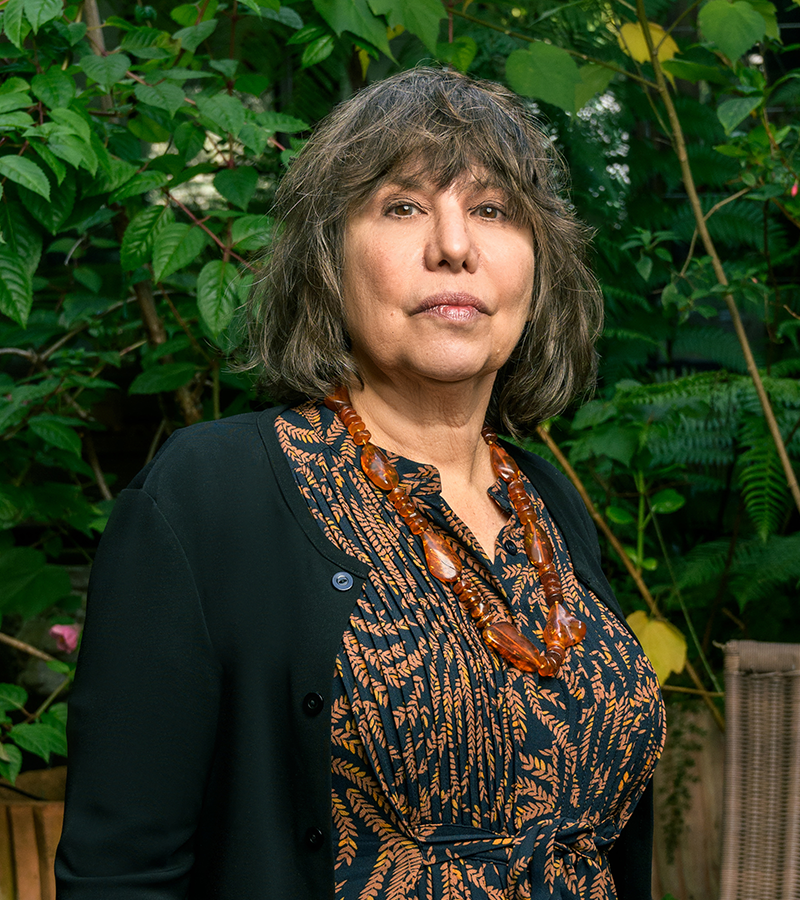
Photo: Winni Wintermeyer
Q: What are the scientific hurdles to understanding causality in human and animal brains? Is this knowledge transferable to AI?
Alison Gopnik: One of the most important things that we all need to do, as thinking creatures, is to learn about cause-and-effect. It’s an important problem for science, really the central problem.
When adults try to solve causal problems in our everyday lives, we mostly rely on information we already have. This includes basic stuff, like if I turn on this tap, water will come out. But in the case of children and scientists, the information they need isn’t already there. They have to acquire it somehow from the patterns they observe out in the world.
How to do that is a problem that any genuinely intelligent system, whether biological or computational, will have to solve — and children seem to be the best at it. So for the last 20-plus years, we’ve been looking at how children solve that problem.
Q: You’re renowned in the field of developmental psychology for the “blicket.” Can you tell us about that?
AG: The blicket detector is a little box that lights up and plays music when you put some objects on top of it, but not if you put on other objects. We ask the child, which one is a
blicket? Can you tell me how it works? Can you make it go? With this very simple machine, we can present children with different patterns of data to see what conclusions they draw.
At first, we’d manipulate the objects for them. But we very quickly saw that the kids always wanted to touch the blickets themselves and try them on the machine. They wanted to play with them. They don’t want to watch, they want to do.
If you think about science, sometimes scientists’ jobs will be to just look at giant databases and try and work out the statistics from them, which is sort of what most of today’s neural network AI systems do. They’re given a giant dataset and asked to pull out the structure. But the gold standard for science is controlled experiments, cause-and-effect stuff that actually manipulates something in the world to find out about how it works.
We’re trying to see if we can look at children’s active
learning — the way they experiment and play and do things out in the world — and give a good computational account of those processes.
“One of the most important things that we all need to do, as thinking creatures, is to learn about cause-and-effect. It’s an important problem for science, really the central problem.”
— Alison Gopnik
Q: An ongoing debate is whether artificial intelligence will be able to perform as well or better than humans on a range of cognitive tasks, achieving what would be called “artificial general intelligence” (AGI). On a scale from one to 10, with one being impossible and 10 being certain, how likely is it that we’ll see AGI within a decade?
AG: From my perspective as a developmental psychologist, I don’t think there’s any such thing as AGI so I’ll say zero.
The more we study children, the more we realize how even the simplest cognitive tasks that we take for granted are incredibly complex. And we don’t really understand how children learn as much as they do, as quickly as they do. I’m skeptical that we’ll solve that problem with artificial agents in anything like 10 years.
That said, AI will get much better at doing specialized tasks. I think their role as cultural technologies is going to be really interesting. We humans will have access to much more information.
For humans, inventions like writing and print and internet search engines let us transmit and access more information. These are all really important technologies that can change the way we function. Large language models are the latest example of such a technology — they summarize and access all the information on the internet. But that’s really different from human intelligence. I think that AI is very far away from achieving that.
DHANYA SRIDHAR
Université de Montréal Canada CIFAR AI Chair, Mila
Dhanya Sridhar develops theory and machine learning methods to study causal questions. Her research focuses on theoretically understanding what causal questions can be answered from observed data and adapting machine learning methods to estimate causal effects. Sridhar combines causality and machine learning in service of AI systems that are robust, adaptive and useful for scientific discovery.
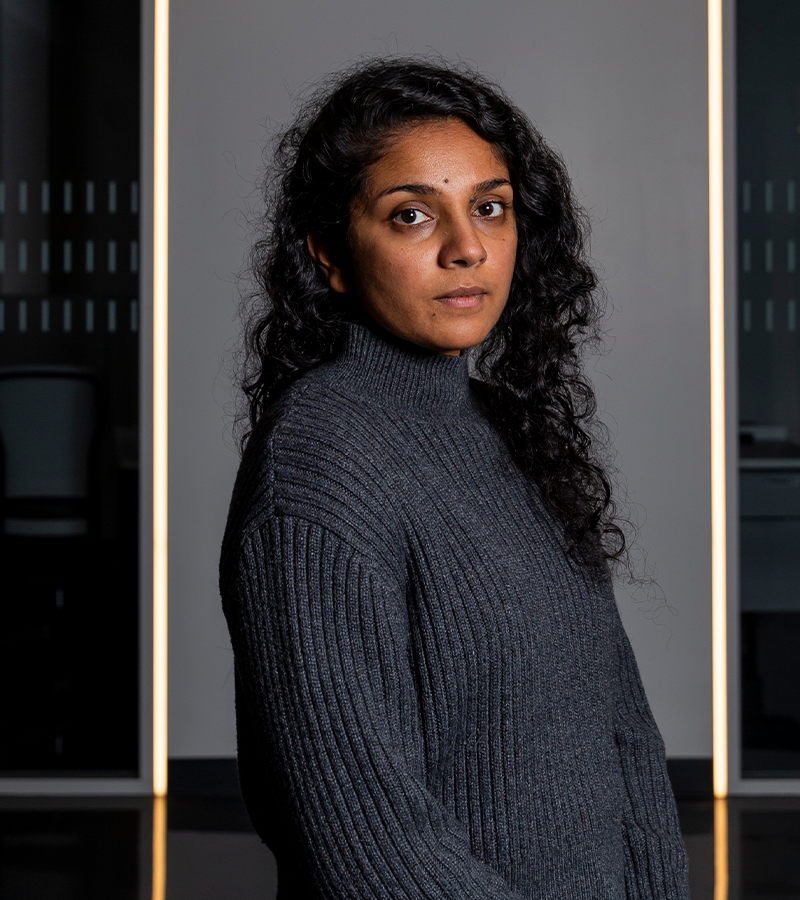
Photo: Dominic Blewett
Q: Why is causality so central to the field of AI?
Dhanya Sridhar: Humans and other organisms seem tuned to notice changes in our environment. We may notice that some variables, when perturbed in a certain way, affect the state of other variables. We especially take notice when this pattern — that variable one affects variable two — reliably occurs no matter what’s happening with other variables in the system. We call that invariance.
When we note such a reproducible pattern of change, it’s convenient to call it a causal relationship. So “cause” is shorthand to describe relationships that seem to be robust and stable, no matter how other parts of the systems change.
Why is keeping track of causal relationships important and interesting for us organisms? It’s because of their invariance. They allow organisms to anticipate outcomes, plan and react accordingly.
In the same vein, causality could be key for AI systems that are making predictions from complex data. Identifying and using causal relationships could help AI systems produce responses that are robust and grounded in reliable patterns.
Q: Given that causality is a really key question in both AI and neuroscience, how can the fields learn and teach each other about causal mechanisms?
DS: My understanding is that humans and many other species are tuned to look for causal patterns. As we interact with the world or as we watch others interact with the world, we take notice when some action seemingly leads to a changed state. If this change occurs in many diverse conditions, we are tuned to recognize it as a sort of causal “rule.”
But in our complex world, the ability to quickly recognize causal patterns can also be a bug: we humans unfortunately incorrectly attribute causation to coincidences. Sometimes an event A happens, followed by an event B and we conclude that A must have caused B. Then, confirmation bias can reinforce this faulty worldview.
When we consider learning algorithms, the question is, are they similarly tuned to noticing patterns that remain invariant across contexts — in other words, that stay the same — and leveraging these patterns to make future predictions? For humans, identifying causal relationships helps us build a model of the world that is highly modular, with many pieces of knowledge that remain the same even as other knowledge changes. Given that large machine learning systems are increasingly trained on multiple data sources that are highly diverse — data that’s necessary to learn causal models — a key question for AI research is figuring out how to encourage learning algorithms to latch onto invariant patterns.
Q: Let’s say we solve this causal machine learning problem. If AI gets really adept at understanding causality, what is at stake? What could the outcome be?
DS: One answer is what we call “out-of-distribution generalization.” This is the idea that we want AI systems to not be too bad at predicting outcomes or making decisions in scenarios they have never encountered before. What is “not too bad”? Well, if AI systems increasingly relied on fairly stable associations when making predictions, these stable relationships are likely to still hold to some extent in new contexts.
Another potential impact if AI systems identified causal knowledge, is avoiding harmful biases. Consider racist stereotypes. Stereotypes can be thought of as associations that are not, in fact, reflective of causal relationships. So if we have systems that prefer to model invariant or causal relationships, they may avoid using stereotypes as shortcuts when making high-stakes decisions.
On a related note, AI systems that use causal relationships could also be far more trustworthy. If I, as a user, were to deploy an AI system in a sensitive domain, I might have more trust in a system that behaves consistently and isn’t sensitive to irrelevant details. Such a guarantee might be especially important knowing that adversarial users will likely try to exploit such sensitivities in models to get them to generate harmful outputs.
“Consider racist stereotypes. Stereotypes can be thought of as associations that are not, in fact, reflective of causal relationships. So if we have systems that prefer to model invariant or causal relationships, they may avoid using stereotypes as shortcuts when making high-stakes decisions.”
— Dhanya Sridhar
Q: Speaking of safety, what is your opinion about whether AI science will lead to AGI in the next decade, on a scale from one to 10?
DS: I’d like to reframe the question as follows: are future AI systems likely to be more robust reasoners than today’s systems? My answer is: it’s not at all a given. The future of AI depends on how well we, as AI researchers, come to understand the biases and shortcuts that learning algorithms are prone to taking. Without better understanding of how modern learning algorithms behave and taking steps to guide them towards robust solutions using some theory about causality, I don’t think we can take for granted that future AI systems will be more robust.
KONRAD KORDING
University of Pennsylvania Program Co-Director, CIFAR Learning in Machines & Brains
Konrad Kording seeks to understand the brain as a computational device. Using deep learning as a means of modeling brain functions, the Kording lab broadly uses data analysis methods, including machine learning, to ask fundamental questions.
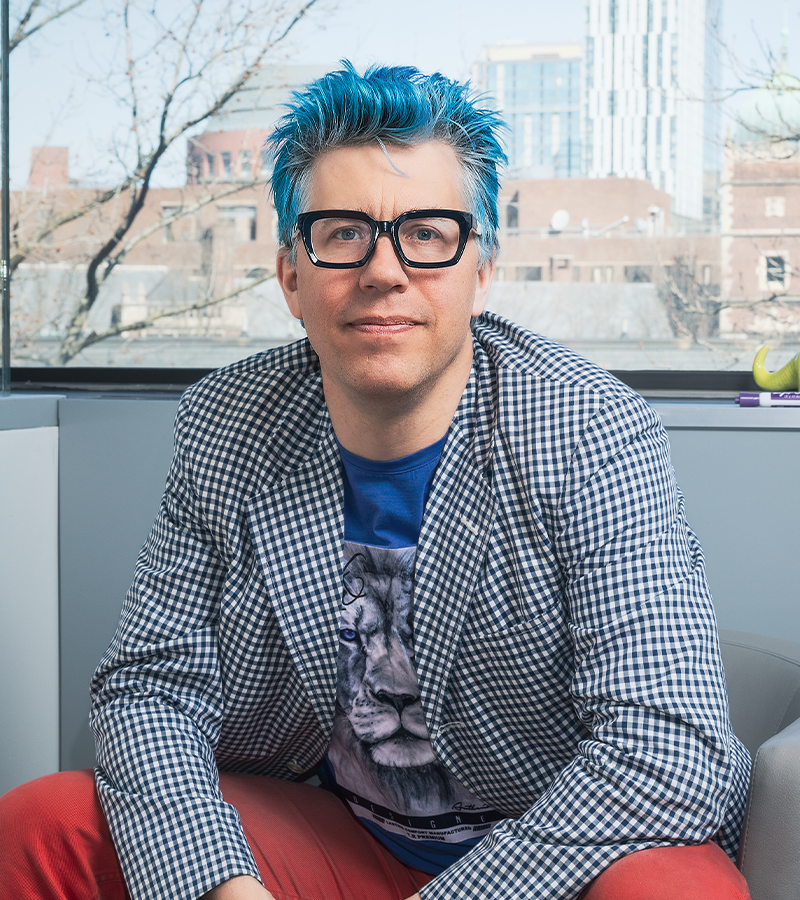
Photo: Colin Lenton
Q: Your work straddles the fields of neuroscience and AI. What are your thoughts on the scientific obstacles to understanding how our brains untangle causality and how we might model that to improve AI?
KK: Every human story, every study you’ll ever read or write about, is a story about cause. It’s always, “How did one thing make another thing happen?” That’s how humans think, it’s central to who we are. But if you ask me how the brain does it, I have to tell you that we have absolutely no clue.
If I ask you how you understand cause and effect in a given situation, you’ll probably say it’s because someone explained it to you. Or maybe you learned it from messing around and trying stuff as a kid. And then there’s the third way, which is through observation. You watched a rock roll down a mountain and damage a tree and now you know that a rock can damage a tree. But we actually have no idea how the brain does any of these things. So making the leap to computation is extremely tricky.
Q: You’ve been working on strategies to use machine learning systems to model causality. Can you tell us a little bit about that work?
KK: Every human has experienced causality in millions of ways. We kick a rock and it hurts. We throw rocks, we carve rocks and we have someone else cut rocks and we have all that experience in all those causal domains. What we wanted to do is take this logic and bring it into a machine learning setting.
We needed a situation where we could do a lot of causal experiments. So we take a simulated microprocessor and we perturb it. We go in with a wire and put some extra current in the transistors and try things. It’s like kicking a rock, but on a transistor level. Then, based on data like time tracers of the transistors, we see if we can predict what happens and what is happening in the processor. We do that in the order of thousands of times, with thousands of interactions in the transistors to train the system. We see if the system can figure out causality on the other side without additional training data.
Q: We’ve seen the videos of robots learning to do mundane things and it often takes a really long time before they get proficient at simple tasks. Why are robots seemingly so hobbled?
KK: Humans are born with a lot of prior knowledge that robots are not born with. Humans seem to naturally understand causal relations. Alison Gopnik’s work shows us in wonderful ways that babies want to understand the world in terms of causality. There is this built-in bias that is not shared by robots and our current AI systems. So we have to force it into them by giving them so much training data that unless they discover how the world causally works, they’re really helpless. And this means we just need so much more data.
The way we think about it as AI researchers is that this need for so much data is basically to make up for that evolutionary advantage that got humans to where we are today.
“What people need to understand about today’s AI systems is that they’re very good sidekicks. They’re just not good heroes.”
— Konrad Kording
Q: On that question of AI’s capacity to rival the human brain: on a scale from one to 10, how likely is it that the field of AI will reach AGI in the next decade?
KK: I’ll say two. Almost everyone in the AI field believes that we are heading towards this. But there is a lot of uncertainty about the timing, some people say five years; others say more like 50. I think people need to understand that AI isn’t at all like humans. It doesn’t think like us. It thinks very, very differently, if it’s even fair to call it thinking. I also have some doubts as to whether it’s even a desirable goal.
In the intelligence of humans, we have encoded the kind of information that makes society stable, even in times of crisis. There is encoded information about how to get unstuck if you’re stuck. And AI systems can’t do those things because crises don’t happen enough for AI. Your genome tells the story of ten thousand droughts and how to survive them. The emotional and social data that humans are hard-wired for goes much deeper than AI could learn from any parent dataset. There is no repository of data for 10,000 crises of humankind.
Then again, there are lots of ways in which AI is already better than you and me and has been for a long time now. It multiplies numbers better and it finds books better and we don’t have any issue with that. So while some people feel that AGI is coming to make us obsolete, I think that instead, it will make the tedious parts of life obsolete.
What people need to understand about today’s AI systems is that they’re very good sidekicks. They’re just not good heroes.
Related Articles
-
CIFAR renews programs in AI, child development and quantum materials
March 13, 2025
-
Rich Sutton honoured with Turing Award for major contributions to AI
March 10, 2025
-
Looking ahead: the future of AI in Canada
March 05, 2025
-
CIFAR funds seven high-risk, high-reward AI projects
March 04, 2025