Learning in Machines & Brains

How do we understand intelligence and build intelligent machines?
Learning in Machines & Brains program draws on neuro- and computer science to investigate how brains and artificial systems become intelligent through learning. The program’s fundamental approach — going back to basic questions rather than focusing on short-term technological advances — has the dual benefit of improving the engineering of intelligent machines and leading to new insights into human intelligence.
IMPACT CLUSTERS
The Learning in Machines & Brains program is part of the following CIFAR Impact Clusters: Decoding Complex Brains and Data and Exploring Emerging Technologies. CIFAR’s research programs are organized into 5 distinct Impact Clusters that address significant global issues and are committed to fostering an environment in which breakthroughs emerge.
RESEARCH AND SOCIETAL IMPACT HIGHLIGHTS
A partnership to advance artificial intelligence research
CIFAR’s Learning in Machines & Brains program has an ongoing partnership with Inria, the French national research institute for digital science and technology. Much like CIFAR, Inria encourages scientific risk-taking and interdisciplinarity and both organizations are leaders in pioneering new approaches to machine learning and AI.
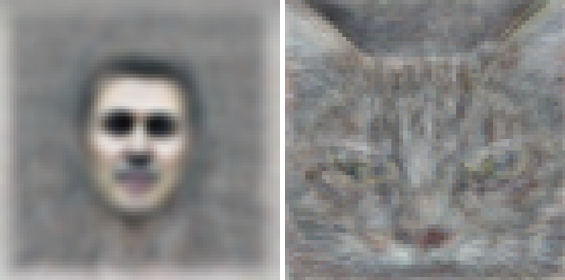
Using AI to learn about the brain
CIFAR Fellow and Canada CIFAR AI Chair Blake Richards (McGill University, Mila) and Associate Fellow Joel Zylberberg (York University) led a workshop with experts from academia, industry and non-profit institutions to discuss the scale, scope, use cases, organizational structures and funding required to build neuro-foundation models, applying recent advances in AI to better understand the brain. These big-scale machine learning models, pre-trained on large quantities of data, can be adapted to new tasks with relatively small amounts of new training data and computational power, and could potentially serve both the neuroscience and neurotechnology communities, while also benefiting machine-learning research.
Bridging the gap between machine learning and human intelligence
Program Co-Director Yoshua Bengio’s (IVADO, Canada CIFAR AI Chair at Mila, Université de Montréal) research group is developing new theories to bridge the gap between current machine-learning techniques and human intelligence. By studying the kind of inductive biases that humans and animals exploit, CIFAR researchers are clarifying the principles that are hypothesized to guide human and animal intelligence, and which could provide inspiration for both AI research and neuroscience. Work continues to develop AI systems that can exhibit flexible out-of-distribution learning and systematic generalization, areas where contemporary machine learning approaches still lag human cognitive abilities.
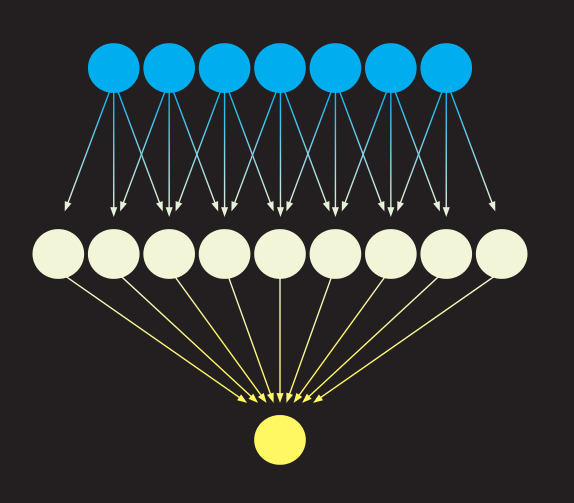
SELECTED PAPERS
Hinton, G. E., Osindero, S. and Teh, Y. (2006). “A fast learning algorithm for deep belief nets.” Neural Computation, 18, pp 1527-1554.
Y. Bengio and P. Lamblin and D. Popovici and H. Larochelle, “Greedy Layer-Wise Training of Deep Networks,” Neural Information Processing Systems Proceedings (2006).
Salakhutdinov, R. and Hinton, G., “Learning a Nonlinear Embedding by Preserving Class Neighbourhood Structure,” Proceedings of the Eleventh International Conference on Artificial Intelligence and Statistics, 412-419 (2007).
Graves, A., Mohamed, A., Hinton, G. E., “Speech Recognition with Deep Recurrent Neural Networks,” 39th International Conference on Acoustics, Speech and Signal Processing, Vancouver (2013).
Yann LeCun, Yoshua Bengio and Geoffrey Hinton. (2015). “Deep Learning.” Nature, 521, pp 436–444. ABSTRACT
Path to Societal Impact
We invite experts in industry, civil society, healthcare and government to join fellows in our Learning in Machines & Brains program for in-depth, cross-sectoral conversations that drive change and innovation.
Social scientists, industry experts, policymakers and CIFAR fellows in the Learning in Machines & Brains program are addressing complex ethical issues in research and training environments and in the implementation of AI.
Areas of focus:
- Exploring existing and future societal implications of AI research.
- Addressing issues in AI research and implementation, including privacy, accountability, and transparency.
Founded
2004
Renewal Dates
2008, 2014, 2019
Partners
Inria
Supporters
Alfred P. Sloan Foundation, RBC Foundation
Interdisciplinary Collaboration
Computer science, including artificial intelligence, deep learning, reinforcement learning
Neuroscience
Bioinformatics
Computational biology
Statistics
Data science
Psychology
CIFAR Contact
Fellows & Advisors
Program Directors
Fellows
Advisors
CIFAR Azrieli Global Scholars
Support Us
CIFAR is a registered charitable organization supported by the governments of Canada and Quebec, as well as foundations, individuals, corporations and Canadian and international partner organizations.